Rupert Menneer, Christos Margadji, Sebastian W. Pattinson
Christos Margadji
PhD Researcher
Computer-aided Manufacturing Group
University of Cambridge, Department of Engineering
Division E, Institute for Manufacturing (IfM)
email: cm2161(at)cam(dot)ac(dot)uk
Bio
I am a 3rd year PhD Researcher with the Computer-aided Manufacturing (CAM) lab of the University of Cambridge supervised by Dr. Sebastian Pattinson. Prior to joining the lab, I obtained an MSc in Artificial Intelligence from Imperial College London, Department of Computing supervised by Pr. Andrew Davison. I also obtained my BEng in Mechanical Engineering from University of Birmingham.My research interest lies in applying artificial intelligence for real world problems, in industrial robots for manufacturing, physics modeling and beyond.
I have friends in industry through a number of internships, including one with the Materials Science Division of Lawrence Livermore National Laboratory (LLNL) in California, USA and one with the Security Division of International Business Machines (IBM) in London, UK.
I was the recipient of scholarships from the Engineering and Physical Sciences Research Council (EPSRC) as well as the Cyprus State Scholarship Foundation.
Selected Projects
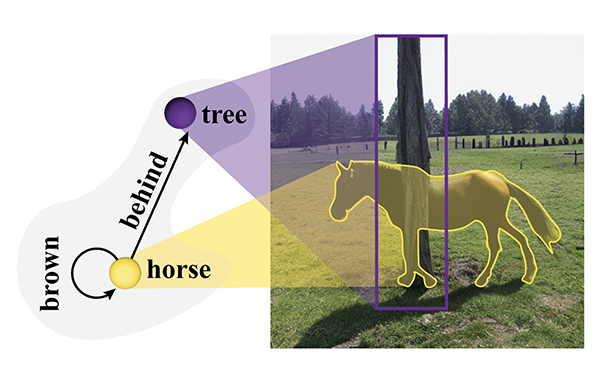
Graph-Conditioned Diffusion for Image Synthesis, 2025,
Rupert Menneer, Christos Margadji, Sebastian W. Pattinson
We introduce a novel method for conditioning diffusion-based image synthesis models with heterogeneous graph data. Existing approaches typically incorporate conditioning variables directly into model architectures, either through cross-attention layers that attend to text latents or image concatenation that spatially restrict generation. However, these methods struggle to handle complex scenarios involving diverse, relational conditioning variables, which are more naturally represented as unstructured graphs. This paper presents Heterogeneous Image Graphs (HIG), a novel representation that models conditioning variables and target images as two interconnected graphs, enabling efficient handling of variable-length conditioning inputs and their relationships. We also propose a magnitude-preserving GNN that integrates the HIG into the existing EDM2 diffusion model using a ControlNet approach. Our approach improves upon the SOTA on a variety of conditioning inputs for the COCO-stuff and Visual Genome datasets, and showcases the ability to condition on graph attributes and relationships represented by edges in the HIG.
Rupert Menneer, Christos Margadji, Sebastian W. Pattinson
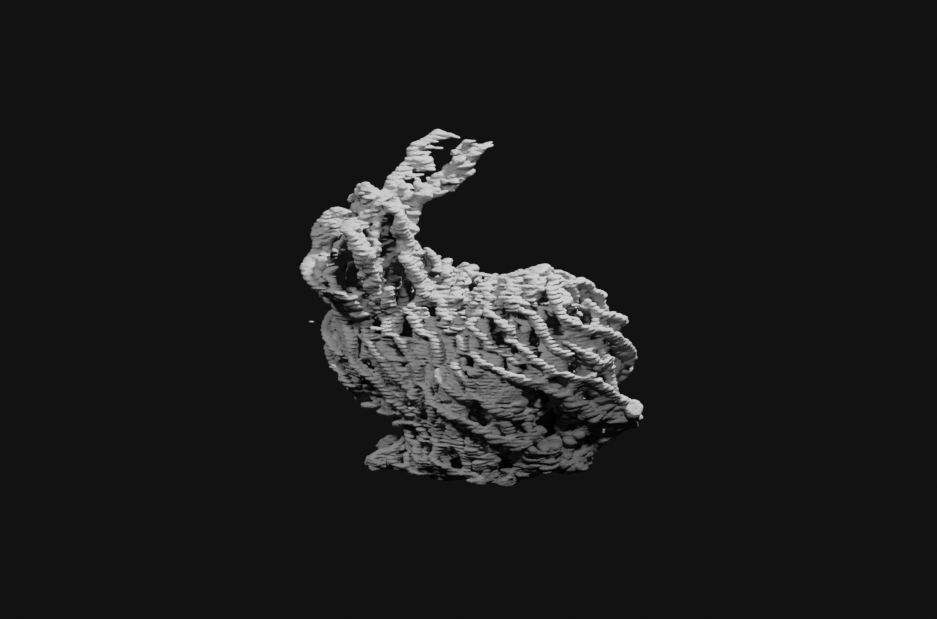
Regularized interpolation in 4D neural fields enables optimization of 3D printed geometries, 2025,
Christos Margadji, Andi Kuswoyo, Sebastian Pattinson.
Neural implicit representations (NIRs) excel at encoding various types of modalities by linking coordinates to signal values, allowing the modelling of diverse dynamic phenomena and complex, high-dimensional data. While proficient at generalizing to resolutions beyond those of the training data, NIRs exhibit unstable interpolation capabilities when supervision is sparse. To address this challenge we introduce Gradient-Driven Interpolation Regularisation (GDIR) which involves computing and minimizing the partial derivative of the NIR's output with respect to sparse coordinates, thereby enabling smooth interpolation between them. Furthermore, we demonstrate that NIRs can be parameterized with manufacturing process parameters to represent the volumetric data of 3D printed parts, allowing reconstruction at previously unseen parameters. Finally, we show that models trained with GDIR can be employed for data-driven optimization to maximize the geometric fidelity between the printed artifact and its computational model. This work contributes to enhancing the robustness and applicability of NIRs in practical domains such as manufacturing.
Christos Margadji, Andi Kuswoyo, Sebastian Pattinson.
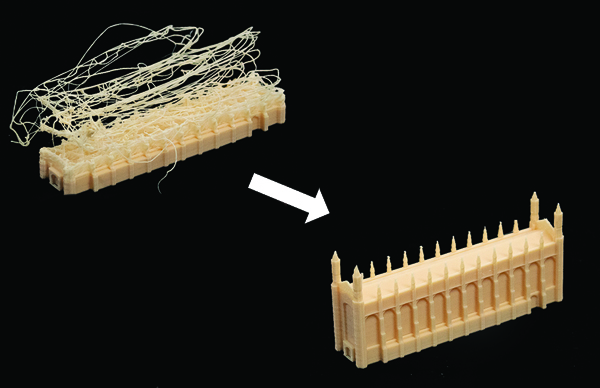
Iterative learning for efficient additive mass production, 2024,
Christos Margadji, Douglas Brion, Sebastian Pattinson.
Material extrusion could enable on-demand production of complex and personalized parts but is limited by low reliability, particularly in higher-volume production. Machine learning-based methods may enhance reliability, but are often themselves insufficiently reliable for use in production. Foundation artificial intelligence models have enabled significant improvements in performance across many tasks. Here, a vision-based control system is reported, coupling active learning and uncertainty awareness with a foundation model to continually learn to build a specific part better. The resulting framework is called Iterative Learning, as it improves performance by learning from its own errors during repeated build cycles of the same part. The iterative learning approach is shown to enable robust error detection and correction while being more space, time and computationally efficient compared to a naive fine-tuning approach. This provides a path showing how foundation models may be adapted to enhance reliability across a wider range of additive manufacturing processes.
Christos Margadji, Douglas Brion, Sebastian Pattinson.
Material extrusion could enable on-demand production of complex and personalized parts but is limited by low reliability, particularly in higher-volume production. Machine learning-based methods may enhance reliability, but are often themselves insufficiently reliable for use in production. Foundation artificial intelligence models have enabled significant improvements in performance across many tasks. Here, a vision-based control system is reported, coupling active learning and uncertainty awareness with a foundation model to continually learn to build a specific part better. The resulting framework is called Iterative Learning, as it improves performance by learning from its own errors during repeated build cycles of the same part. The iterative learning approach is shown to enable robust error detection and correction while being more space, time and computationally efficient compared to a naive fine-tuning approach. This provides a path showing how foundation models may be adapted to enhance reliability across a wider range of additive manufacturing processes.
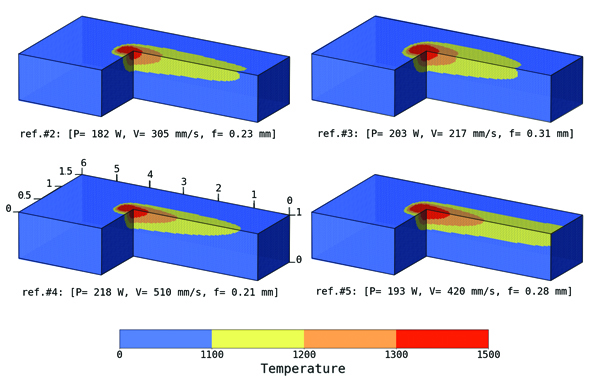
Physics-informed neural networks in additive manufacturing, 2022,
Christos Margadji, Andrew Davison, Paul Hooper.
Laser Powder Bed Fusion is revolutionising the space and defence industries. However, its multi-physics and non-deterministic nature often render the process unstable, leading to inconsistencies in the quality of manufactured parts. Computer vision and Machine Learning can provide in-situ monitoring for flaw detection, but real-time flaw localisation remains intractable. The industry is shifting towards digital twins, that should enable further understanding of the system’s dynamics for physics-informed decisions. One of the main pillars of DT are mechanistic models, frequently expressed by partial differential equations (PDE) and traditionally solved using finite methods. This forms a bottleneck: Given the fractal resolutions needed for multi-physics LPBF modelling, prohibitive solving times are required. In this report, a parameterised Physics-informed Neural Network (PINN) is trained without any data and in a self-supervised manner to approximate the heat diffusion PDE in the context of an LPBF setup. During inference it can instantly predict thermal profiles (3D structure) and steady-state melt pool characteristics (length, width, depth, area, volume) for any combination of laser focus, power and velocity. Other use cases are also discussed. The model takes into consideration the non-linear thermal-dependent properties of the assumed material, that being 316L stainless-steel. Its reliability is verified by comparing its outputs with theoretical ground-truth data.
Christos Margadji, Andrew Davison, Paul Hooper.
Laser Powder Bed Fusion is revolutionising the space and defence industries. However, its multi-physics and non-deterministic nature often render the process unstable, leading to inconsistencies in the quality of manufactured parts. Computer vision and Machine Learning can provide in-situ monitoring for flaw detection, but real-time flaw localisation remains intractable. The industry is shifting towards digital twins, that should enable further understanding of the system’s dynamics for physics-informed decisions. One of the main pillars of DT are mechanistic models, frequently expressed by partial differential equations (PDE) and traditionally solved using finite methods. This forms a bottleneck: Given the fractal resolutions needed for multi-physics LPBF modelling, prohibitive solving times are required. In this report, a parameterised Physics-informed Neural Network (PINN) is trained without any data and in a self-supervised manner to approximate the heat diffusion PDE in the context of an LPBF setup. During inference it can instantly predict thermal profiles (3D structure) and steady-state melt pool characteristics (length, width, depth, area, volume) for any combination of laser focus, power and velocity. Other use cases are also discussed. The model takes into consideration the non-linear thermal-dependent properties of the assumed material, that being 316L stainless-steel. Its reliability is verified by comparing its outputs with theoretical ground-truth data.
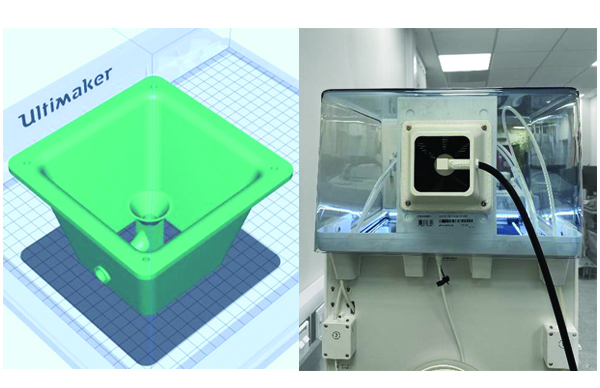
Characterising nanoparticle emissions released from 3D printing, 2021,
Christos Margadji, Omid Doustdar, Jose Herreros.
Molten material used for heat-assisted material extrusion undergoes thermal decomposition, in the process releasing airborne particles that may be toxic for humans. We evaluate the particle number concentrations formed within the build chamber during printing with various materials, using a scanning mobility particle sizer and a custom-made isokinetic sampling probe. The effect of multi-material printing has also been investigated, while also assessing the effectiveness of the proposed particle mitigation solution. The thermal profile of the selected materials was also obtained using a TGA. The results indicate that while specific materials are widely regarded as dangerous to work with, there are other less known, yet more volatile, that can cause up to six times higher particle concentrations. It is also evident that while Mono-Extrusion print emissions reach their peak during the warm-up phase, Dual-Extrusion prints release emissions at a steady-state magnitude near that very same peak throughout the whole print. The filter was found to be effective; however, the ventilation of the system inadequate. In general, the study suggests that more research should be put into the emissions of all available materials before reaching the public and that more intense abatement methods should be implemented.
Christos Margadji, Omid Doustdar, Jose Herreros.
Molten material used for heat-assisted material extrusion undergoes thermal decomposition, in the process releasing airborne particles that may be toxic for humans. We evaluate the particle number concentrations formed within the build chamber during printing with various materials, using a scanning mobility particle sizer and a custom-made isokinetic sampling probe. The effect of multi-material printing has also been investigated, while also assessing the effectiveness of the proposed particle mitigation solution. The thermal profile of the selected materials was also obtained using a TGA. The results indicate that while specific materials are widely regarded as dangerous to work with, there are other less known, yet more volatile, that can cause up to six times higher particle concentrations. It is also evident that while Mono-Extrusion print emissions reach their peak during the warm-up phase, Dual-Extrusion prints release emissions at a steady-state magnitude near that very same peak throughout the whole print. The filter was found to be effective; however, the ventilation of the system inadequate. In general, the study suggests that more research should be put into the emissions of all available materials before reaching the public and that more intense abatement methods should be implemented.